We describe a guilt-by-association system that can be used to rank networked entities by their suspiciousness. We demonstrate the algorithm on a suite of data sets generated by a terrorist-world simulator developed to support a DoD program. Each data set consists of thousands of entities and some known links between them. The system ranks truly malicious entities highly, even if only relatively few are known to be malicious ex ante. When used as a tool for identifying promising data-gathering opportunities, the system focuses on gathering more information about the most suspicious entities and thereby increases the density of linkage in appropriate parts of the network. We assess performance under conditions of noisy prior knowledge of maliciousness. Although the levels of performance reported here would not support direct action on all data sets, the results do recommend the consideration of network-scoring techniques as a new source of evidence for decision making. For example, the system can operate on networks far larger and more complex than could be processed by a human analyst. This is a follow-up study to a prior paper; although there is a considerable amount of overlap, here we focus on more data sets and improve the evaluation by identifying entities with high scores simply as an artifact of the data acquisition process.
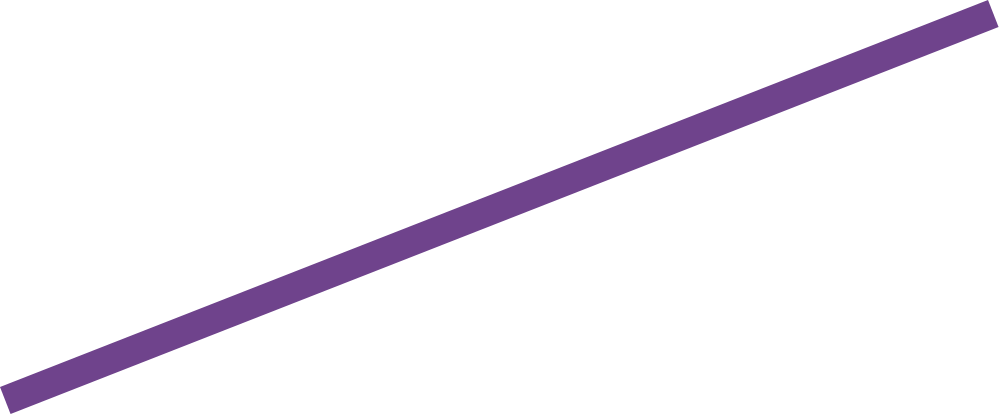
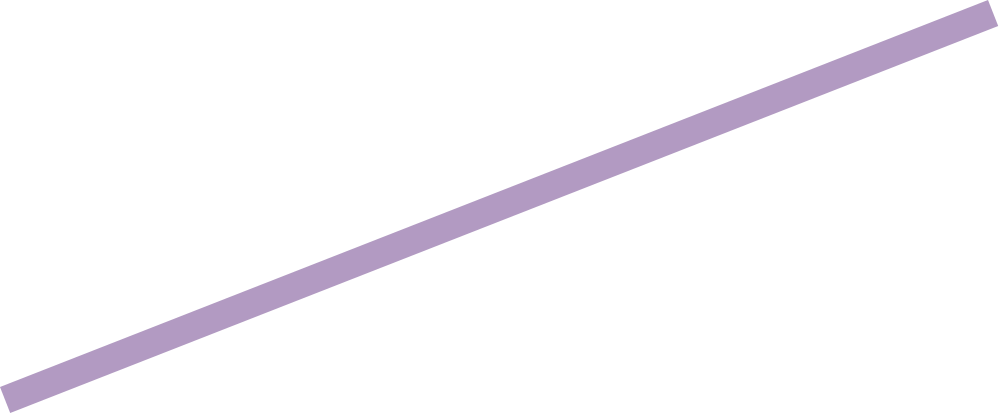


Suspicion Scoring of Networked Entities Based on Guilt-by-Association, Collective Inference, and Focused Data Access
- Sofus Macskassy
- Foster Provost
- Venue: Annual Conference of the North American Association for Computational Social and Organizational Science (NAACSOS), 2005
- 2005
- Type: Additional Conference Paper