Learning general rules is a basic goal of many concept learning systems. In a 1989 paper, Holte, Acker, and Porter pointed out that this bias toward generality had resulted in a problem with small disjuncts. The problem they discussed was that small disjuncts had high rates of misclassification, and that it was difficult to eliminate the error-prone small disjuncts without affecting the performance of other disjuncts. We describe a real domain based on NYNEX MAX, an expert system that diagnoses the local loop in a telephone network. We demonstrate with two inductive learning systems that a range of disjunct sizes is important for this domain despite the relatively high error rates of the small disjuncts. We conclude that the need for smaller disjuncts is a major reason that it is difficult to learn from errorful data in this domain.
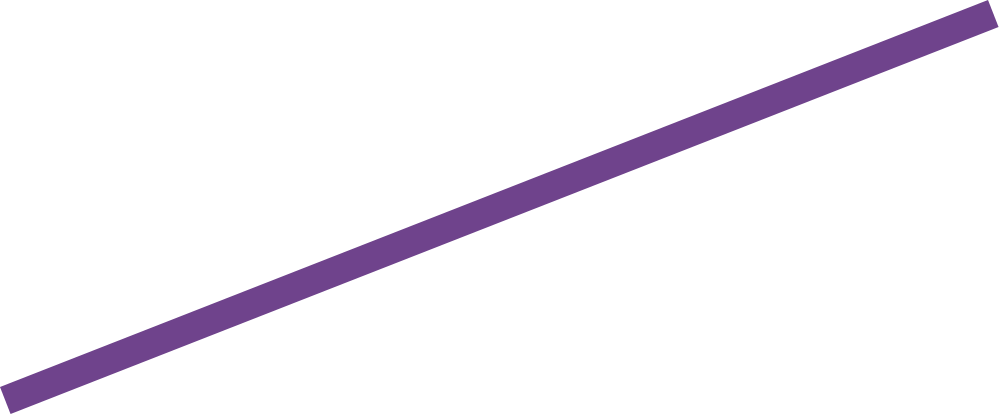
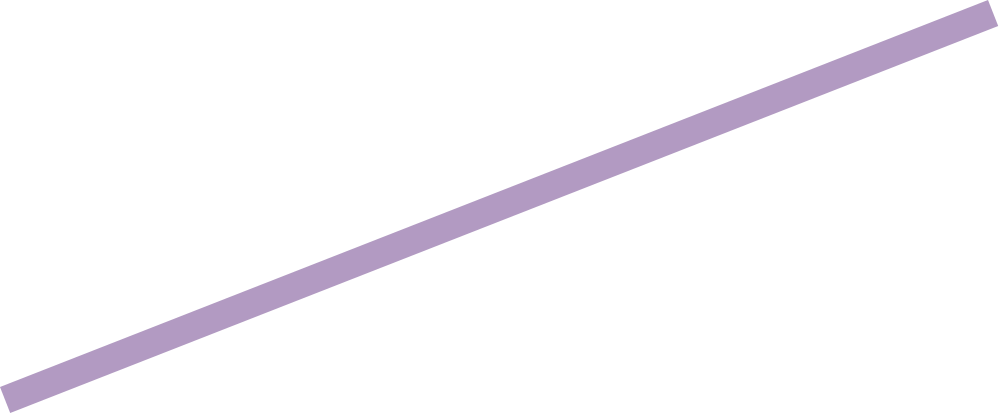


Small Disjuncts in Action: Learning to Diagnose Errors in the Telephone Network Local Loop
- Andrea Danyluk
- Foster Provost
- Venue: Tenth International Conference on Machine Learning (ICML93)
- 1993
- Type: Selected Conference Paper
- Acceptance rates: 27%