Predictive models are often employed to decide actions in interactive online systems. For example, ads are selectively served to users who are modeled as being inclined to purchase the product being advertised. News feed items are populated based on a model of the user’s interests. A common consequence of these predictive models is the creation of a spurious correlation, or confounding, between the action and its desired outcome. In the above examples, the targeted users are likely to buy the product or find the news item regardless of the intervention. This presents a challenge for measuring the true impact of these systems. Here we present a novel framework for estimating causal effects that relies on neither randomized experiments nor adjusting for the potentially explosive number of variables used in predictive models. We propose the identification and instrumentation of events that mediate the effect of the action. When the effect of an action depends on a mediating event that is not subject to the same con founders, the problem of causal estimation is greatly simplified. We demonstrate this approach in display advertising using ad viewability as a natural experiment that mediates the impact of served ads. Approximately 45% of display ad impressions never make it into a viewable portion of the user’s browser. We show that an analysis based on ad viewability can massively reduce the amount of bias in estimating campaign lift. We integrate the use of negative controls as well as the identification and adjustment for residual confounding to further reduce the bias in estimated lift to less than 10%. A system using these techniques is deployed to monitor the daily causal impact of many large-scale advertising campaigns.
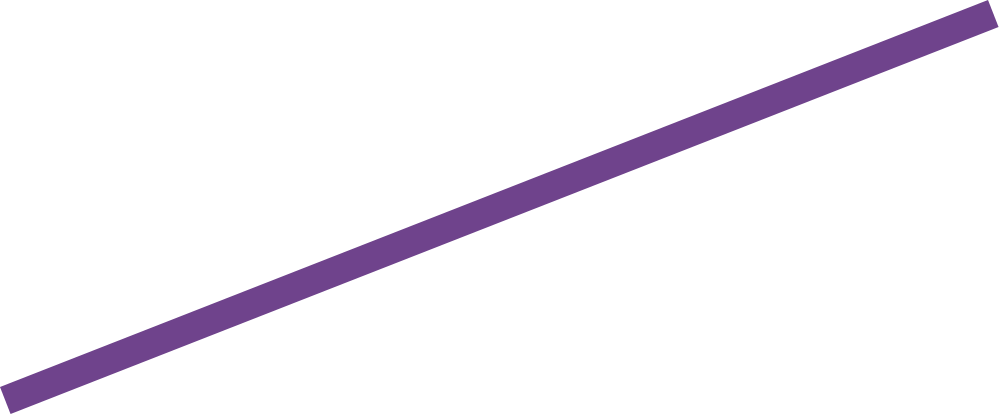
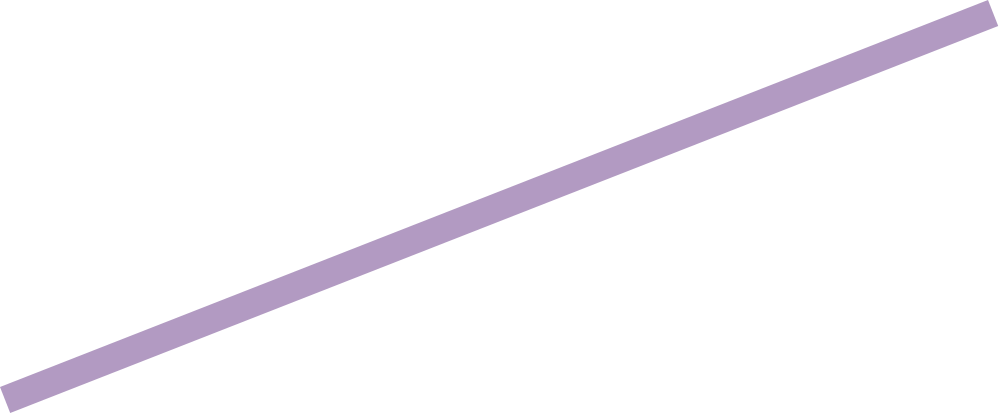


Measuring Causal Impact of Online Actions via Natural Experiments: Application to Display Advertising
- Daniel Hill
- Alan Hubbard
- Robert Moakler
- Foster Provost
- Kiril Tsemekhman
- Vadim Tsemekhman
- Venue: Twenty-first ACM SIGKDD International Conference on Knowledge Discovery and Data Mining (KDD 2015)
- 2015
- Type: Selected Conference Paper