The data describing resolutions to telephone network local loop troubles,” from which we wish to learn rules for dispatching technicians, are notoriously unreliable. Anecdotes abound detailing reasons why a resolution entered by a technician would not be valid, ranging from sympathy to fear to ignorance to negligence to management pressure. In this paper, we describe four different approaches to dealing with the problem of\bad” data in order first to determine whether machine learning has promise in this domain, and then to determine how well machine learning might perform. We then o er evidence that machine learning can help to build a dispatching method that will perform better than the system currently in place.
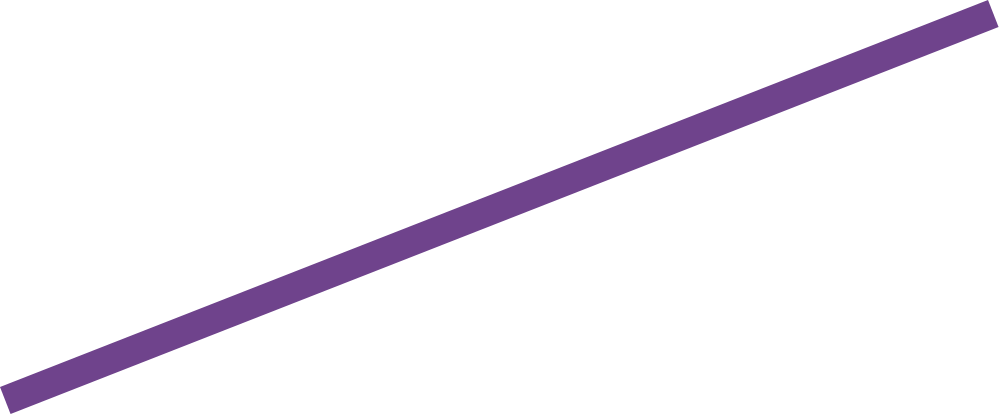
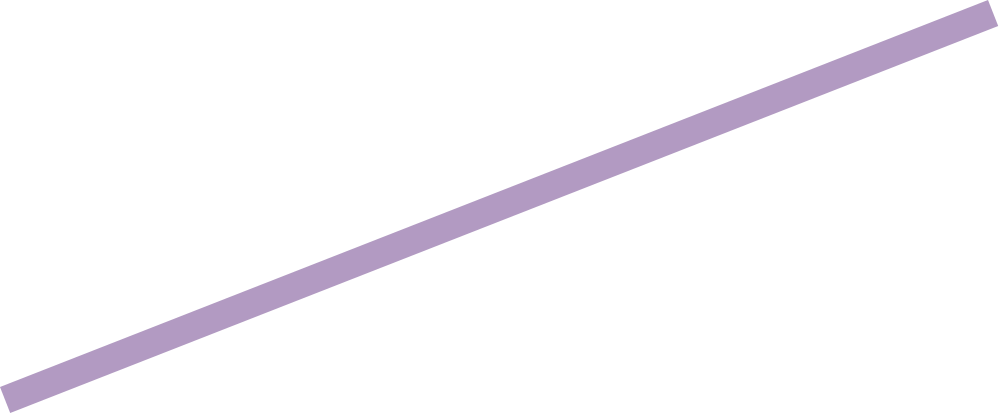


Learning from Bad Data
- Andrea Danyluk
- Foster Provost
- Venue: ML-95 Workshop on Applying Machine Learning in Practice
- 1995
- Type: Other Workshop/Symposium Paper