Research on scalable machine learning algorithms has gained a considerable amount of traction since the exponential growth in data assets during the past decades. Many Big Data applications resort to somewhat “simple” data modelling techniques due to the computational constraints associated with more complex models. Simple models, while being very efficient to estimate, often fail to capture some of the finer details of more complex data sets. In this manuscript, we explore the idea that complex large scale classification can be tractable using a process of iterative refining. In such a process, we focus on non-linearities of the data only after having first found an approximate linear model. This knowledge is then incorporated into the nonlinear model implicitly, allowing the non-linear model to focus on important parts of the data after a rough first estimation. This in turn reduces overall training time and allows for a richer model representation, eventually leading to more predictive power.
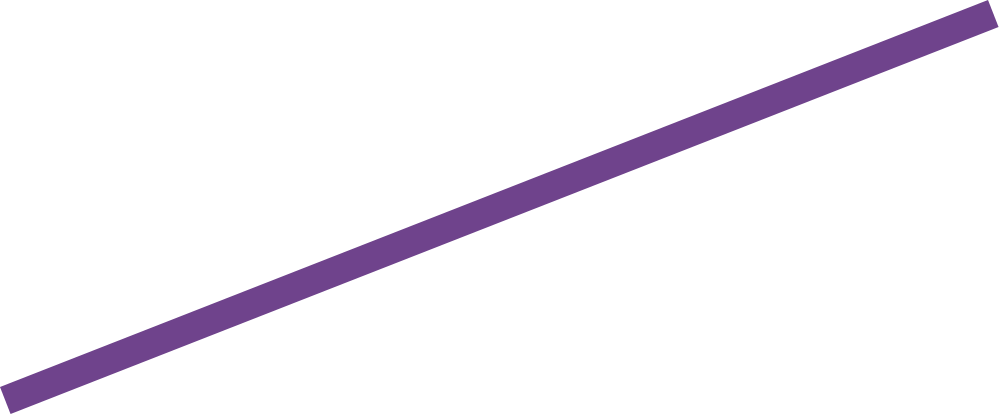
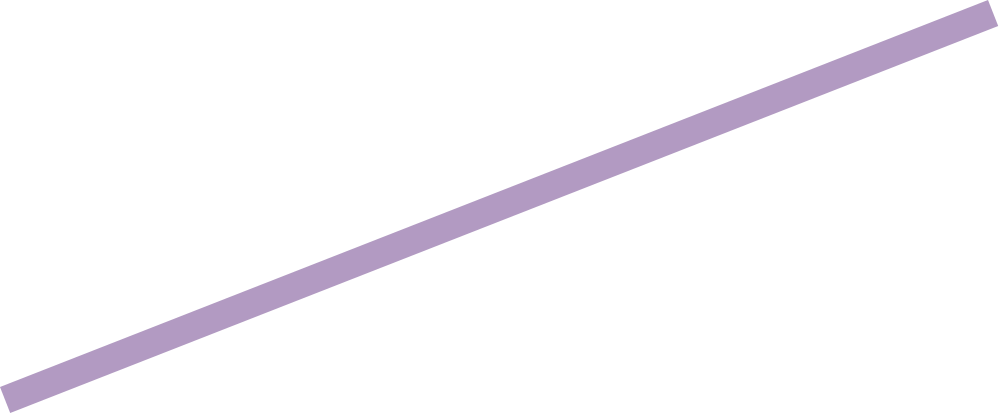


Iteratively Refining SVMs using Priors
- Theodoros Evgeniou
- Enric Junque de Fortuny
- David Martens
- Foster Provost
- Venue: IEEE International Conference on Big Data (IEEE BigData 2015)
- 2015
- Type: Selected Conference Paper