Extreme class skew is a hurdle in many machine learning tasks. In such skewed settings, traditional methods for procuring labeled examples, including random sampling and active learning, are often ineffective—they struggle to find representative minority examples. The framework of Dual Supervision, which incorporates feature-based background information into traditional supervised learning, provides one avenue to combat this problem. However, active learning for feature information (feature labeling), like active learning, is often not resilient to extreme class skew. In this work, we present an alternative to active feature labeling, Guided Feature Labeling. In this paradigm, human domain experts are tasked with finding class indicative features given a description of a class. This work explores different data acquisition costs, and demonstrates that under certain conditions, Guided Feature Labeling does indeed offer high performance models at a far lower budget than complementary active labeling approaches.
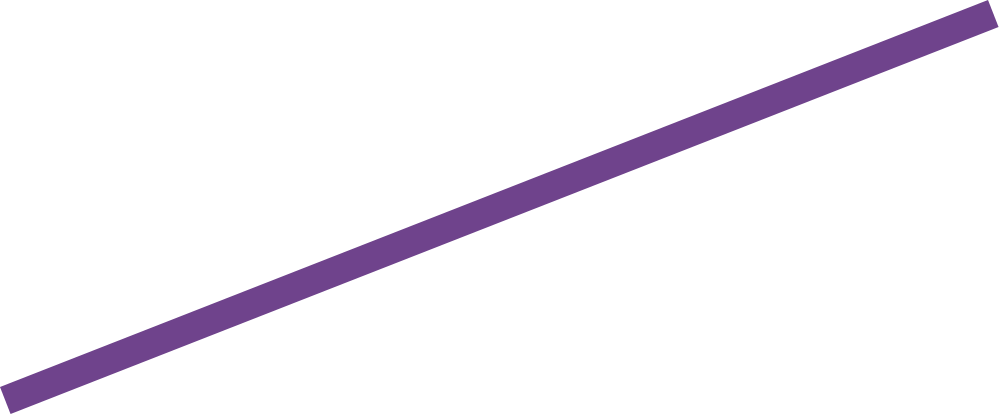
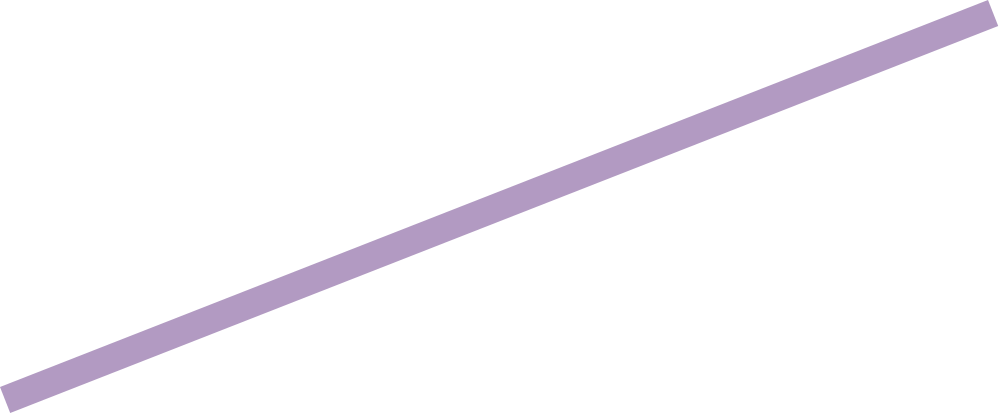


Guided Feature Labeling for Budget-Sensitive Learning Under Extreme Class Imbalance
- Josh Attenberg
- Prem Melville
- Foster Provost
- Venue: ICML-2010 Workshop on Budgeted Learning
- 2010
- Type: Other Workshop/Symposium Paper