In this paper, I present issues and results pertaining to goal-directed inductive machine learning, in particular, inductive learning that takes into account the cost of the errors made when the learned concept description is used. Previous work introduced the notion that learning programs should be able to take as input different policies, so that they can learn under different pragmatic considerations. This paper shows that inductive learning can trade off classification accuracy for a reduction in cost, when the learning program uses a cost function to evaluate its learned knowledge. In particular, I discuss costs related to risks, in the classic mushroom and breast-cancer domains, and monetary costs in a domain of diagnosing errors in the local loop of the telephone network.
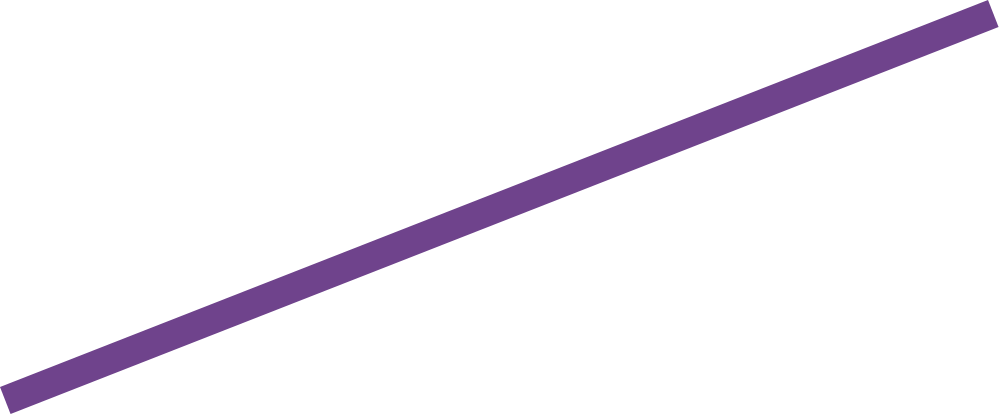
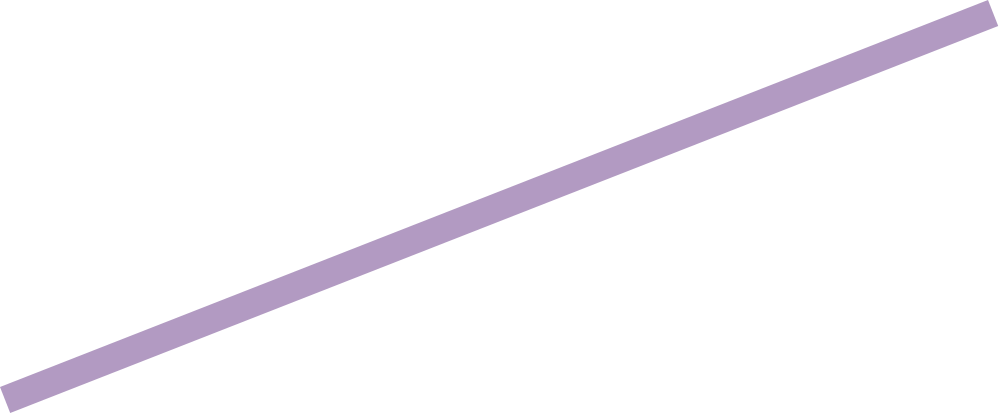


Goal-Directed Inductive Learning: Trading off Accuracy for Reduced Error Cost
- Foster Provost
- Venue: AAAI Spring Symposium on Goal-Directed Learning
- 1994
- Type: Other Workshop/Symposium Paper