In the United States financial firms have the regulatory obligation to monitor the communications of their employees (eg, emails, chats, phone calls) in order to detect misconduct. Some forms of misconduct are illegal activities (eg, insider trading, bribery) while others are policy violations (eg, improper security practices, or inappropriate language use). Traditionally, firms have deployed relatively simple rule-based systems for employee surveillance. Such systems generate many false positive alerts and are hard to adapt to the changing environment. Recently, firms have attempted to improve their systems by transitioning from the rule-based techniques to statistical machine learning approaches. However, they still treat the problem of misconduct detection as a single-document classification problem. We present an approach that focuses on actors, connections among actors, and on cases of misconduct. Furthermore, we highlight the importance of having a “human-in-the-loop” approach, where humans are both guided by and guide the system at the same time, in order to detect malfeasance faster and to adapt to changing environments. We also discuss how humans can play a key role for detecting shortcomings of existing machine-learning based malfeasance-detection systems. Our multifaceted approach has been developed and tested in real environments within both massive and smaller financial institutions, and we discuss practical constraints and lessons learned.
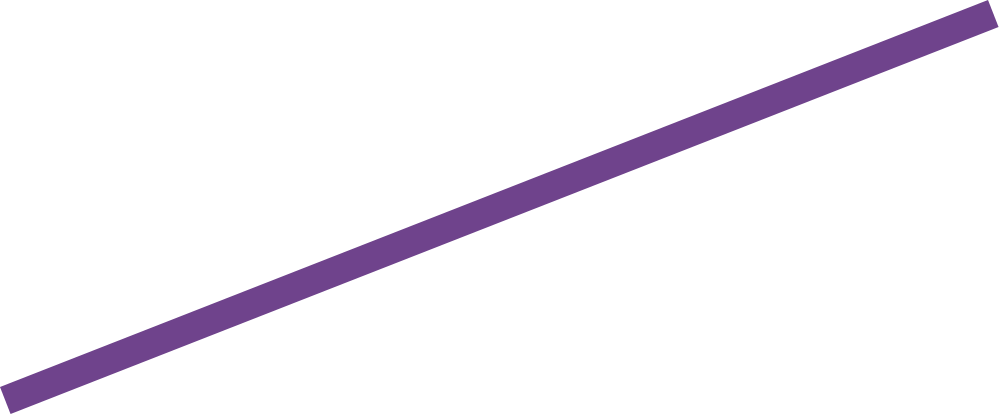
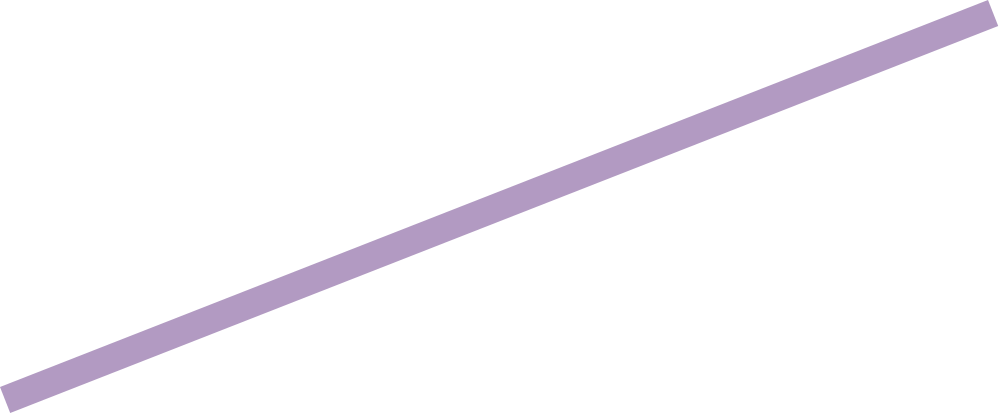


Detecting Employee Misconduct and Malfeasance
- Josh Attenberg
- Jennifer Chin
- Chathra Hendahewa
- Panagiotis Ipeirotis
- Foster Provost
- Abe Stanway
- Bernando Suryanto
- Bharath Vivekananda Swamy
- George Valkanas
- Venue: MIS2: Misinformation and Misbehavior Mining on the Web, 2018, Marina Del Rey, CA, USA
- 2018
- Type: Other Workshop/Symposium Paper