For many supervised learning tasks it is very costly to produce training data with class labels. Active learning acquires data incrementally, at each stage using the model learned so far to help identify especially useful additional data for labeling. Existing empirical active learning approaches have focused on learning classifiers. However, many applications require estimations of the probability of class membership, or scores that can be used to rank new cases. We present a new active learning method for class probability estimation (CPE) and ranking. BOOTSTRAP-LV selects new data for labeling based on the variance in probability estimates, as determined by learning multiple models from bootstrap samples of the existing labeled data. We show empirically that the method reduces the number of data items that must be labeled, across a wide variety of data sets. We also compare BOOTSTRAP-LV with UNCERTAINTY SAMPLING, an existing active learning method designed to maximize classification accuracy. The results show that BOOTSTRAP-LV dominates for CPE. Surprisingly it also often is preferable for accelerating simple accuracy maximization.
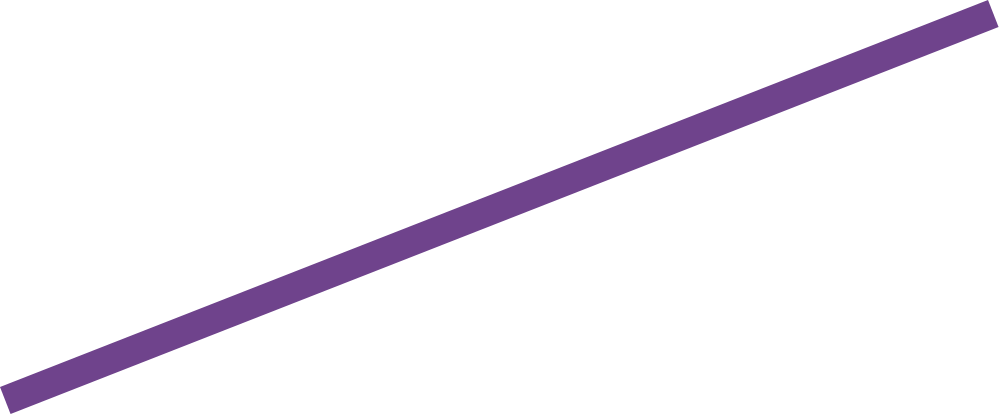
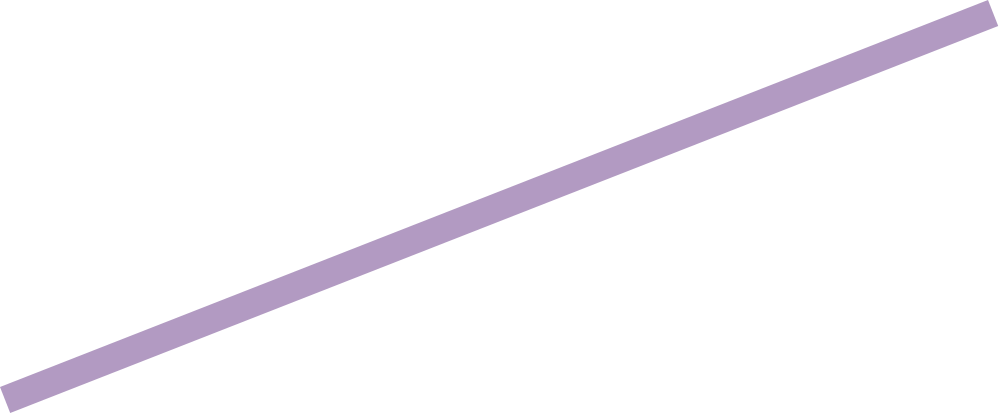


Active Learning for Class Probability Estimation and Ranking
- Foster Provost
- Maytal Saar-Tsechansky
- Venue: Seventeenth International Joint Conference on Artificial Intelligence (IJCAI-01)
- 2001
- Type: Selected Conference Paper
- Acceptance rates: 24%