This paper addresses the classification of linked entities. We introduce a relational vector (VS) model (in analogy to the VS model used in information retrieval) that abstracts the linked structure, representing entities by vectors of weights. Given labeled data as background knowledge training data, classification procedures can be defined for this model, including a straightforward, “direct” model using weighted adjacency vectors. Using a large set of tasks from the domain of company affiliation identification, we demonstrate that such classification procedures can be effective. We then examine the method in more detail, showing that as expected the classification performance correlates with the- relational auto correlation of the data set. We then turn the tables and use the relational VS scores as a way to analyze/visualize the relational auto correlation present in a complex linked structure. The main contribution of the paper is to introduce the relational VS model as a potentially useful addition to the toolkit for relational data mining. It could provide useful constructed features for domains with low to moderate relational auto correlation; it may be effective by itself for domains with high levels of relational auto correlation, and it provides a useful abstraction for analyzing the properties of linked data.
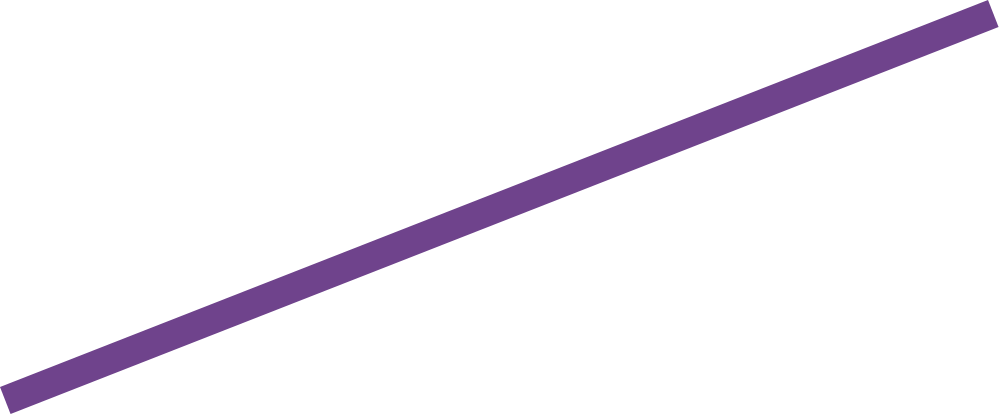
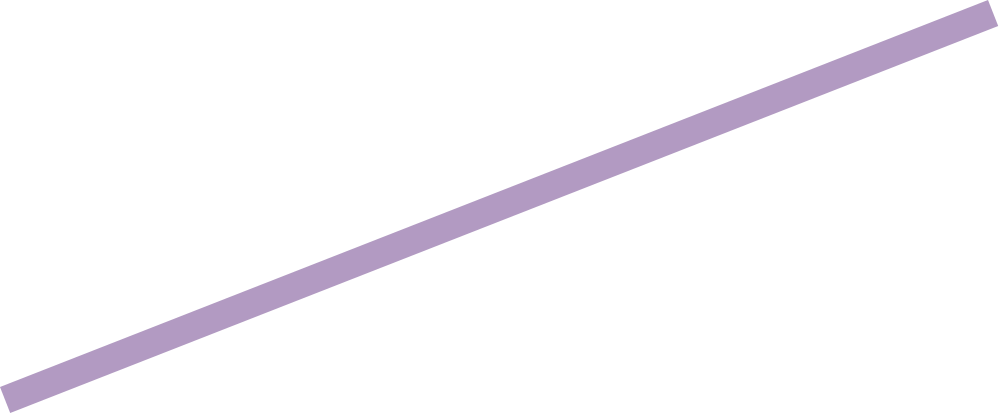


The Relational Vector-Space Model and Industry Classification
- Abraham Bernstein
- Scott Clearwater
- Foster Provost
- Venue: IJCAI-2003 Workshop on Learning Statistical Models from Relational Data
- 2003
- Type: Other Workshop/Symposium Paper