This paper demonstrates that “social network collaborative filtering” (SNCF), wherein user-selected like-minded alters are used to make predictions, can rival traditional user-to-user collaborative filtering (CF) in predictive accuracy. Using a unique data set from an online community where users rated items and also created social networking links specifically intended to represent like-minded “allies” we use SNCF and traditional CF to predict ratings by net-worked users. We find that SNCF using generic “friend” alters is moderately worse than the better CF techniques, but outperforms benchmarks such as by-item or by-user average rating; generic friends often are not like-minded. However, SNCF using “ally” alters is competitive with CF. These results are significant because SNCF is tremendously more computationally efficient than traditional user-user CF and may be implemented in large-scale web commerce and social networking communities. It is notoriously difficult to distinguish the contributions of social influence (where allies influence users) and “social” selection (where users are simply effective at selecting like-minded people as their allies). Nonetheless, comparing similarity over time, we do show no evidence of strong social influence among allies or friends.
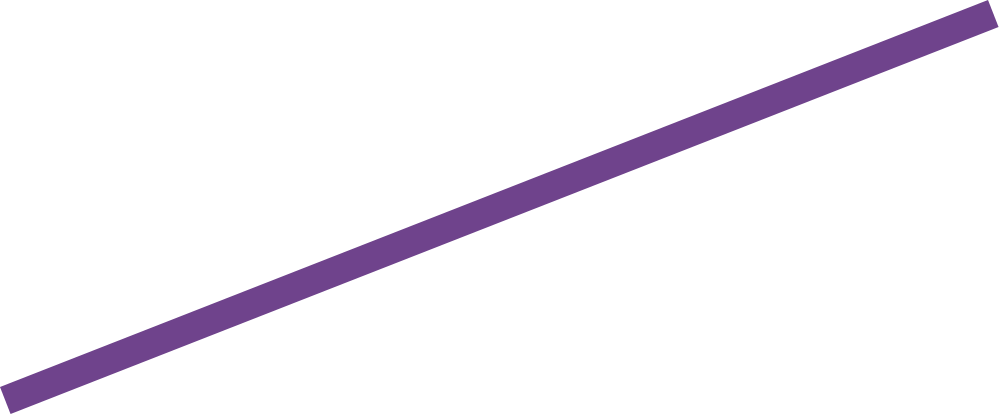
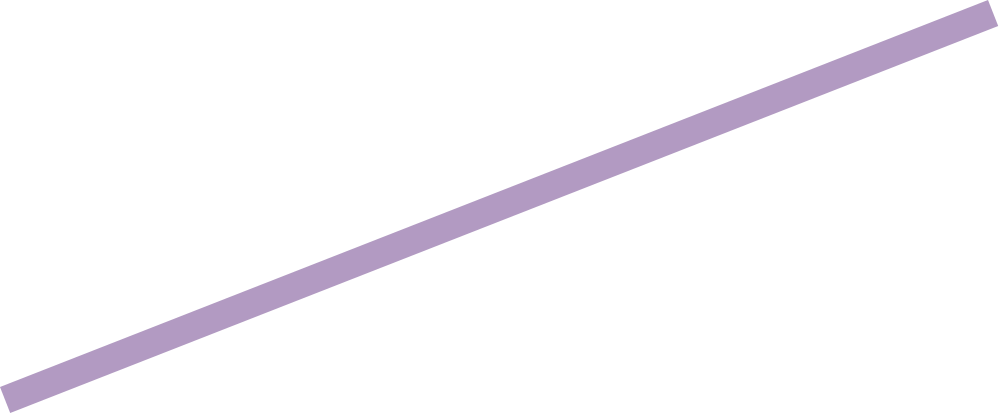


Social Network Collaborative Filtering
- Foster Provost
- Dennis Wilkinson
- Rong Zheng
- Venue: CeDER- 8-08. Center for Digital Economy Research, Stern School of Business, New York University
- 2008
- Type: Other Working Paper