This paper reports on a preliminary empirical study comparing methods for collaborative filtering (CF) using explicit consumers’ social networks. As user-generated social networks become increasingly important and visible in technology-mediated consumer interactions, we can begin to ask how the rich associated information can be used to improve inference. Theories from social psychology have long discussed that social relationships are likely to connect similar people. If the social similarity is in line with the recommendation task, the social network may provide a small, dense set of “recommenders” for CF. To our knowledge this is the first study to show effects of social-network information for estimating purchase behavior with CF. We examine a data set of consumers that contains a social network of consumer-selected friends, as well as their purchases from a large online retailer. We examine two ways to incorporate social-network information into CF: using proximity in the social network to modify the traditional CF, and using the social network to restrict the set of recommenders selected. The results show that social network proximity does not seem to improve recommendations. On the other hand, CF with social-network members selected as recommenders predicts purchases far better than CF with the recommenders not socially connected.
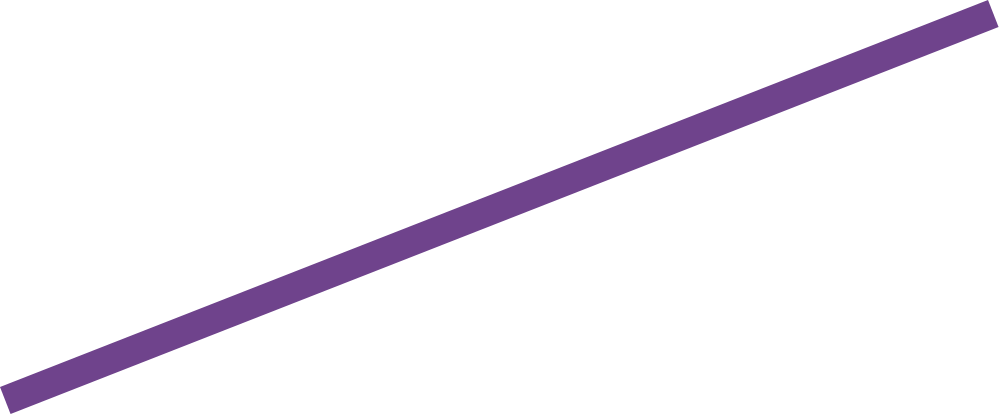
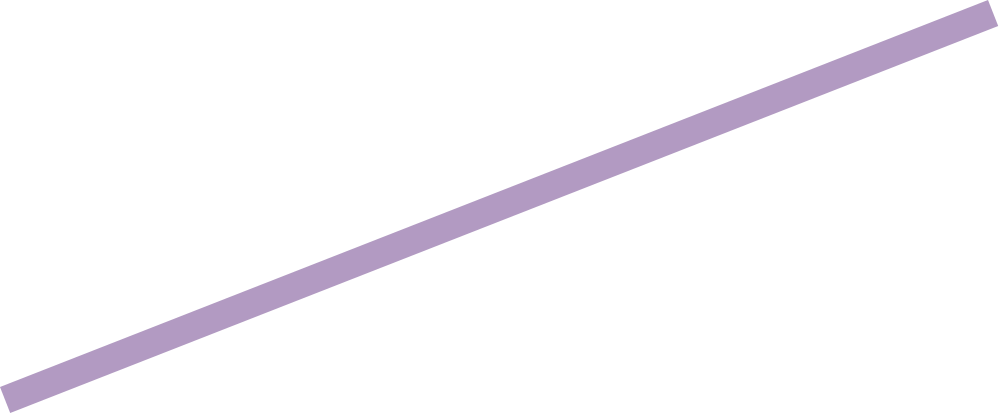


Social Network Collaborative Filtering: Preliminary Results
- Anindya Ghose
- Foster Provost
- Rong Zheng
- Venue: The Sixth Workshop on e-Business (WeB 2007)
- 2007
- Type: Other Workshop/Symposium Paper