This paper is about constructing confidence bands around ROC curves. We first introduce to the machine learning community three band-generating methods from the medical field, and evaluate how well they perform. Such confidence bands represent the region where the “true” ROC curve is expected to reside, with the designated confidence level. To assess the containment of the bands we begin with a synthetic world where we know the true ROC curve—specifically, where the class-conditional model scores are normally distributed. The only method that attains reasonable containment out-of-the-box produces non-parametric, “fixed-width” bands (FWBs). Next we move to a context more appropriate for machine learning evaluations: bands that with a certain confidence level will bound the performance of the model on future data. We introduce a correction to account for the larger uncertainty, and the widened FWBs continue to have reasonable containment. Finally, we assess the bands on 10 relatively large benchmark data sets. We conclude by recommending these FWBs, noting that being non-parametric they are especially attractive for machine learning studies, where the score distributions (1) clearly are not normal, and (2) even for the same data set vary substantially from learning method to learning method.
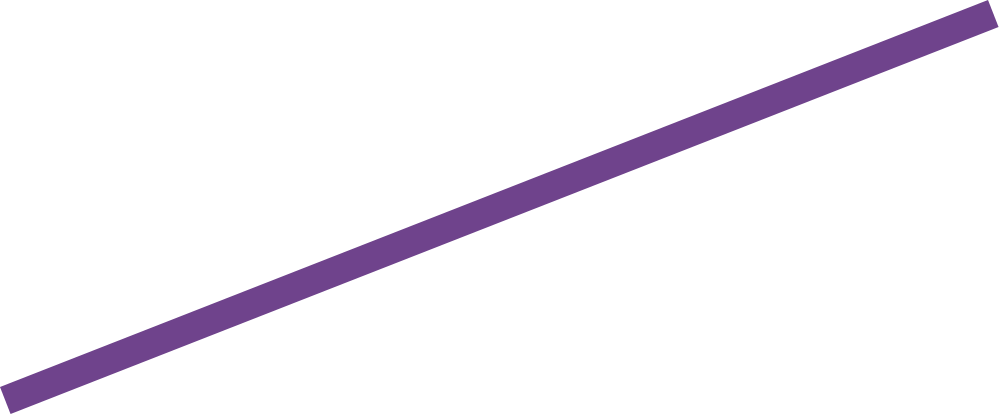
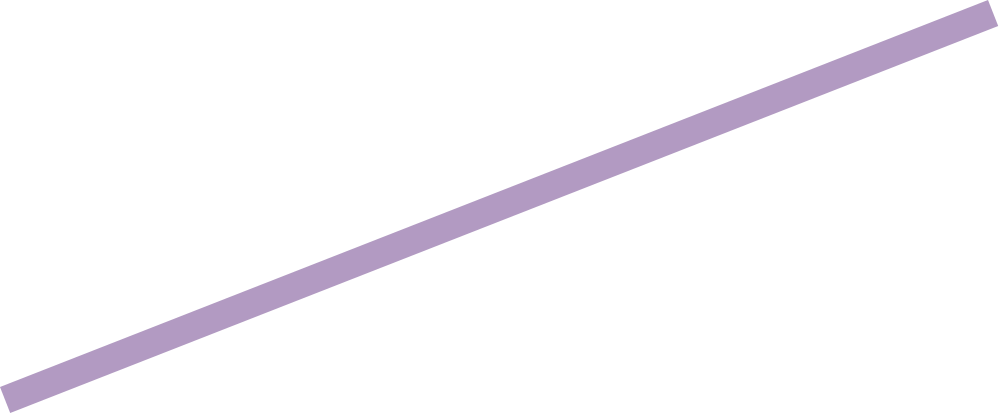


ROC Confidence Bands: An Empirical Evaluation
- Sofus Macskassy
- Foster Provost
- Saharon Rosset
- Venue: 22nd International Conference on Machine Learning (ICML-2005)
- 2005
- Type: Selected Conference Paper
- Acceptance rates: 27%