Training with too much data can lead to substantial computational cost. Furthermore, the creation, collection, or procurement of data may be expensive. Unfortunately, the minimum sufficient training-set size seldom can be known a priority. We describe and analyze several methods for progressive sampling—using progressively larger samples as long as model accuracy improves. We explore several notions of efficient progressive sampling, including both methods that are asymptotically optimal and those that take into account prior expectations of appropriate data size. We then show empirically that progressive sampling indeed can be remarkably efficient.
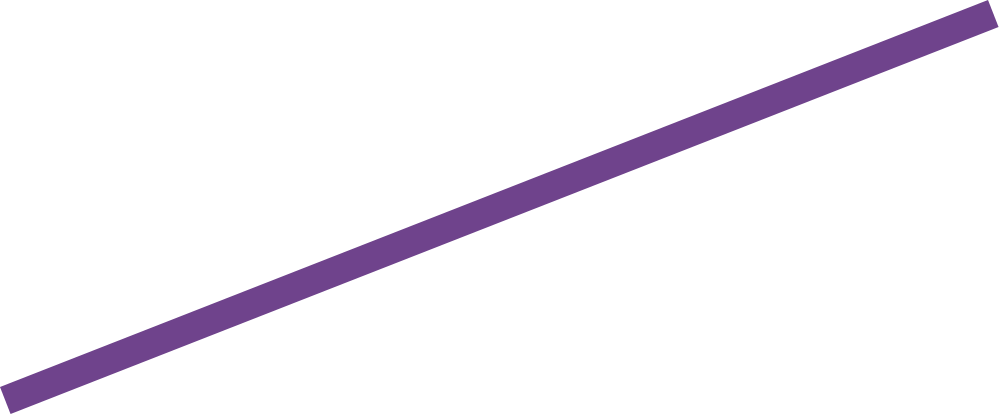
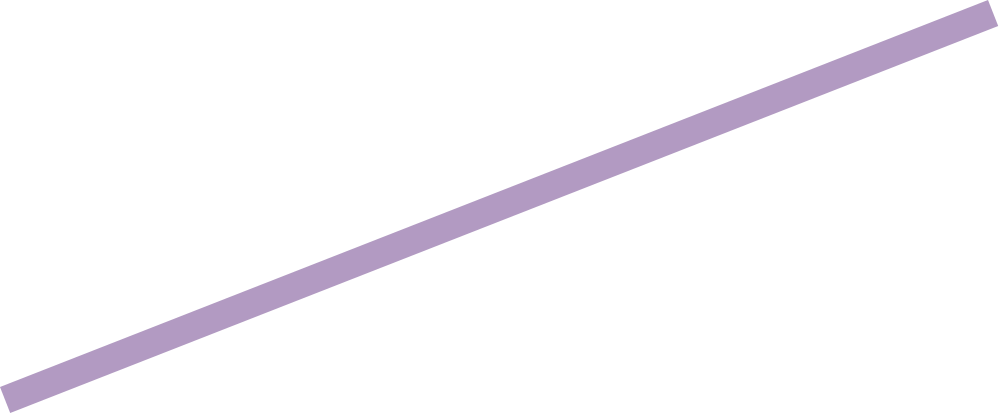


Progressive Sampling
- David Jensen
- Tim Oates
- Foster Provost
- Venue: H. Liu and H. Motoda (eds.), Instance Selection and Construction, A Data Mining Perspective, Kluwer Academic Publishers
- 2000
- Type: Book Chapter & Other Publication