Most video advertising campaigns today are still evaluated based on aggregate demographic audience metrics, rather than measures of individual impact or even individual demographic reach. To fit in with advertisers’ evaluations, campaigns must be optimized toward validation by third-party measurement companies, which act as “oracles” in assessing ground truth. However, information is only available from such oracles in aggregate, leading to a setting with incomplete ground truth. We explore methods for building probabilistic classification models using these aggregate data. If they perform well, such models can be used to create new “engineered” segments that perform better than existing segments, in terms of lift and/or reach. We focus on the setting where companies already have machinery in place for high-performance predictive modeling from traditional, individual-level data. We show that model building, evaluation, and selection can be reliably carried out even with access only to aggregate ground truth data. We show various concrete results, highlighting confounding aspects of the problem, such as the tendency for pre-existing “in-target” segments actually to comprise biased sub-populations, which has implications both for campaign performance and modeling performance. The paper’s main results show that these methods lead to engineered segments that can substantially improve lift and/or reach—as verified by a leading third party oracle. For example, for lifts of 2-3X, segment reach can be increased to 57 times that of comparable, pre-existing segments.
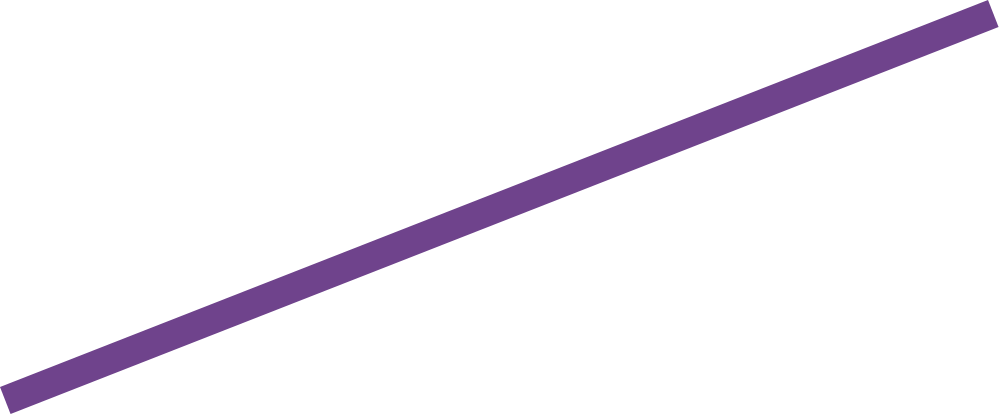
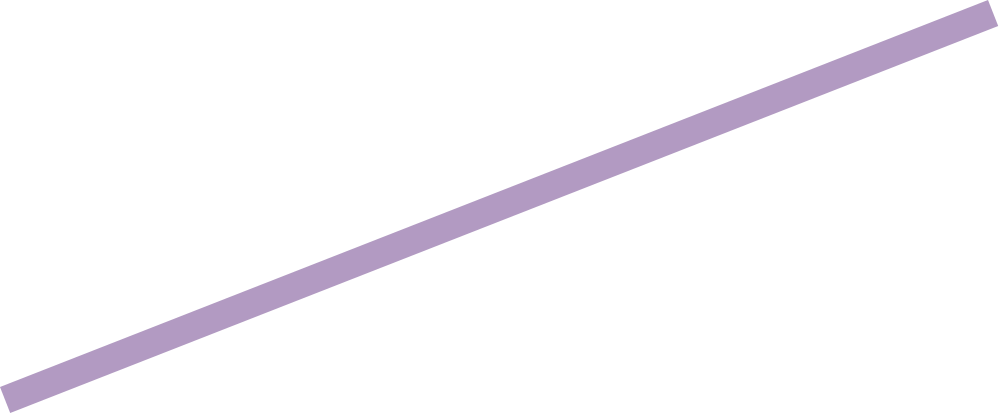


Pleasing the Advertising Oracle: Probabilistic Prediction from Sampled, Aggregated Ground Truth
- Brian Dalessandro
- Claudia Perlich
- Foster Provost
- Melinda Han Williams
- Venue: Eighth International Workshop on Data Mining for Online Advertising (ADKDD 2014).
- 2014
- Type: Other Workshop/Symposium Paper