This paper describes and evaluates privacy-friendly methods for extracting quasi-social networks from browser behavior on user-generated content sites, for the purpose of finding good audiences for brand advertising (as opposed to click maximizing, for example). Targeting social-network neighbors resonates well with advertisers, and on-line browsing behavior data counter intuitively can allow the identification of good audiences anonymously. Besides being one of the first papers to our knowledge on data mining for on-line brand advertising, this paper makes several important contributions. We introduce a framework for evaluating brand audiences, in analogy to predictive-modeling holdout evaluation. We introduce methods for extracting quasi-social networks from data on visitations to social networking pages, without collecting any information on the identities of the browsers or the content of the social-network pages. We introduce measures of brand proximity in the network, and show that audiences with high brand proximity indeed show substantially higher brand affinity. Finally, we provide evidence that the quasi-social network embeds a true social network, which along with results from social theory offers one explanation for the increases in audience brand affinity.
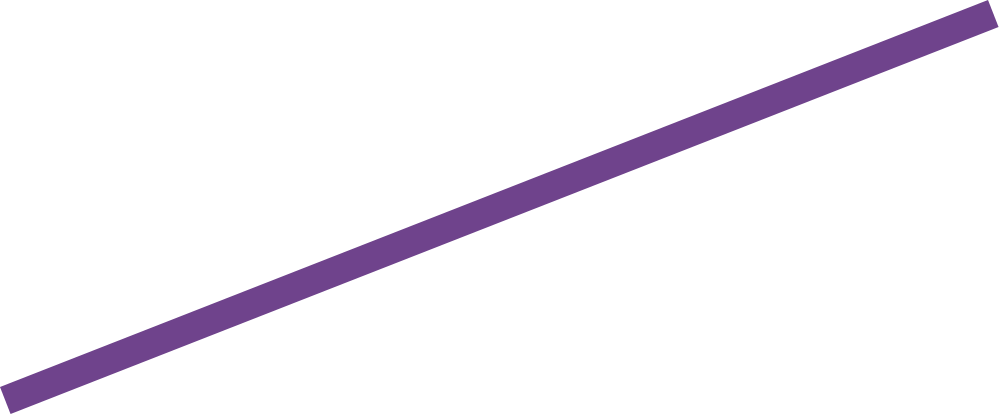
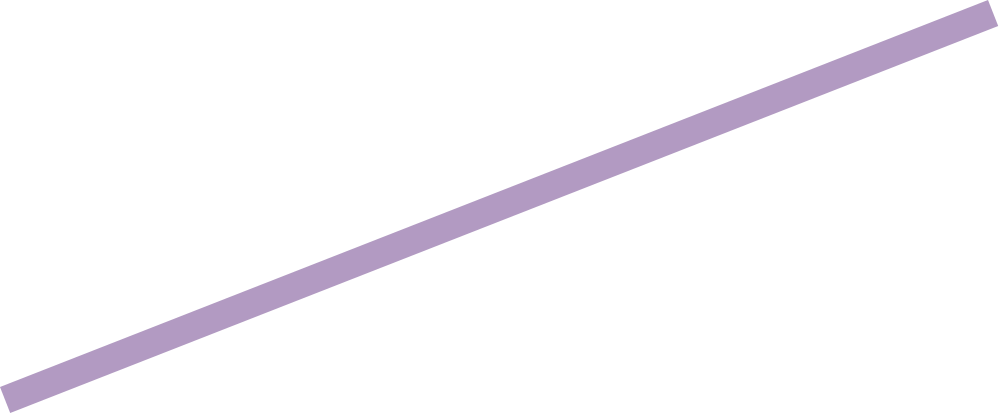


Audience Selection for On-line Brand Advertising: Privacy-Friendly Social Network Targeting
- Brian Dalessandro
- Rod Hook
- Alan Murray
- Foster Provost
- Xiaohan Zhang
- Venue: Fifteenth ACM SIGKDD International Conference on Knowledge Discovery and Data Mining (KDD-2009)
- 2009
- Type: Selected Conference Paper