In many applications we would like to draw inferences about entities that are interconnected in complex networks. For example, calls, emails, IM, and web pointers link people into huge social networks. However, traditional statistical and machine learning classification methods assume that entities are independent of each other. I start by discussing various applications of “classification” (scoring) in networked data, from fraud detection to counter terrorism to network-based marketing. I then discuss four characteristics of networked data that allow improvements– sometimes substantial–over traditional classification: (i) models can take into account “guilt by association,” (ii) inference can be performed “collectively,” whereby inferences on linked entities mutually reinforce each other, (iii) characteristics of linked entities can be incorporated in models, and (iv) models can incorporate specific identifiers, such as the identities of particular individuals, to improve inference. I present results demonstrating the effectiveness of these techniques.
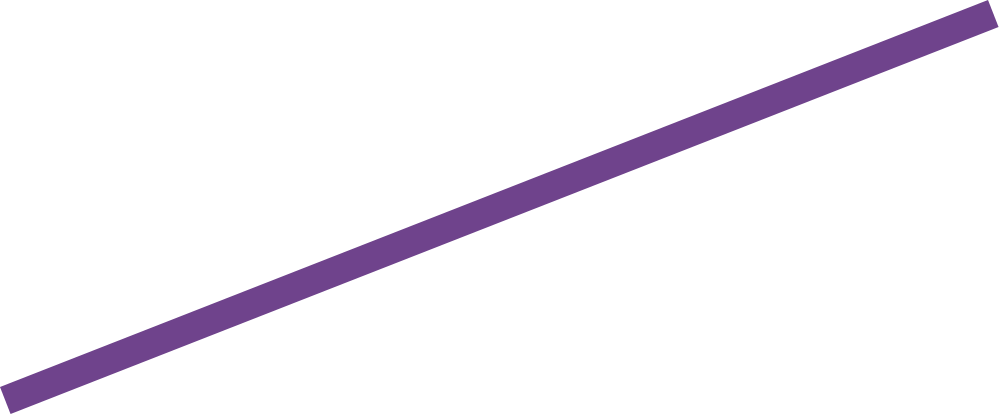
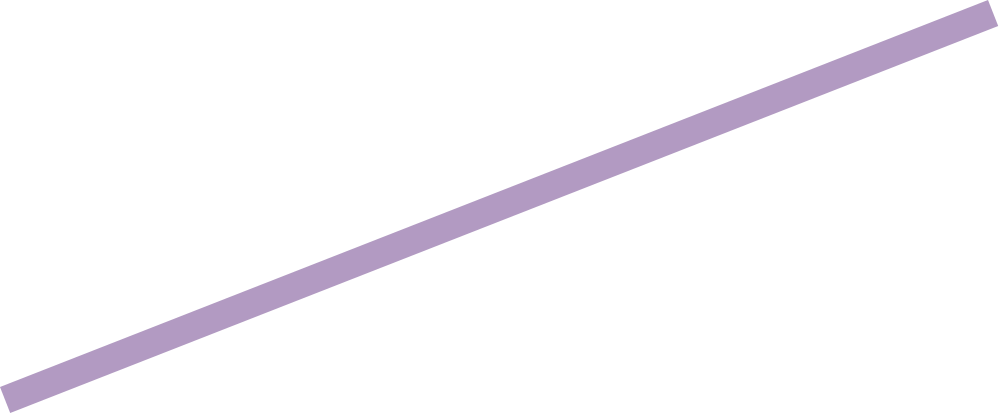

