Online systems promise to improve advertisement targeting via the massive and detailed data available. However, there often is too few data on exactly the outcome of interest, such as purchases, for accurate campaign evaluation and optimization (due to low conversion rates, cold start periods, lack of instrumentation of offline purchases, and long purchase cycles). This paper presents a detailed treatment of proxy modeling, which is based on the identification of a suitable alternative (proxy) target variable when data on the true objective is in short supply (or even completely nonexistent). The paper has a two-fold contribution. First, the potential of proxy modeling is demonstrated clearly, based on a massive-scale experiment across 58 real online advertising campaigns. Second, we assess the value of different specific proxies for evaluating and optimizing online display advertising, showing striking results. The results include bad news and good news. The most commonly cited and used proxy is a click on an ad. The bad news is that across a large number of campaigns, clicks are not good proxies for evaluation or for optimization: clickers do not resemble buyers. The good news is that an alternative sort of proxy performs remarkably well: observed visits to the brand’s website. Specifically, predictive models built based on brand site visits—which are much more common than purchases—do a remarkably good job of predicting which browsers will make a purchase. The practical bottom line: evaluating and optimizing campaigns using clicks seems wrongheaded; however, there is an easy and attractive alternative—use a well-chosen site-visit proxy instead.
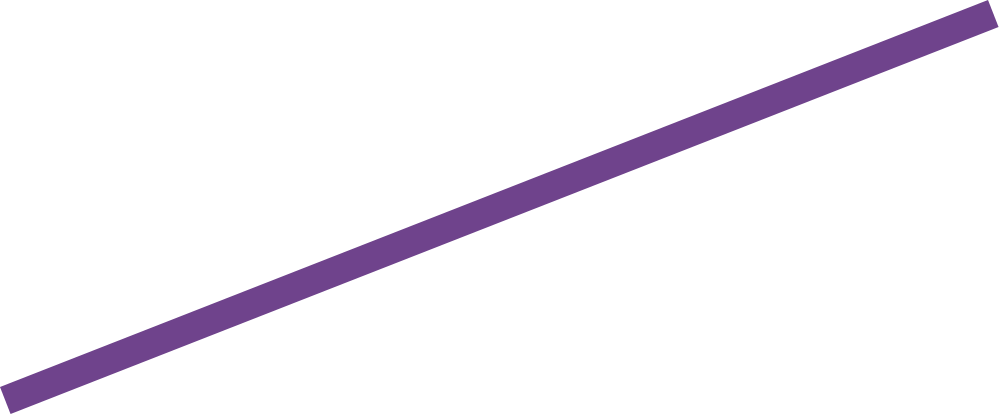
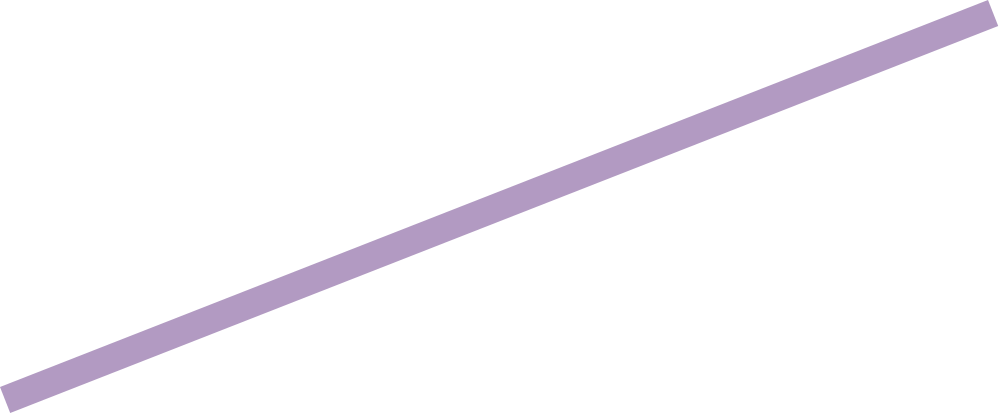


Evaluating and Optimizing Online Advertising: Forget the Click, but there are Good Proxies
- Brian Dalessandro
- Rod Hook
- Claudia Perlich
- Foster Provost
- Venue: Big Data
- 2015
- Type: Journal Article