This paper describes the automatic design of methods for detecting fraudulent behavior. Much of the design is accomplished using a series of machine learning methods. In particular, we combine data mining and constructive induction with more standard machine learning techniques to design methods for detecting fraudulent usage of cellular telephones based on profiling customer behavior. Specifically, we use a rule learning program to uncover indicators of fraudulent behavior from a large database of cellular calls. These indicators are used to create profilers, which then serve as features to a system that combines evidence from multiple profilers to generate high-confidence alarms. Experiments indicate that this automatic approach performs nearly as well as the best hand-tuned methods for detecting fraud.
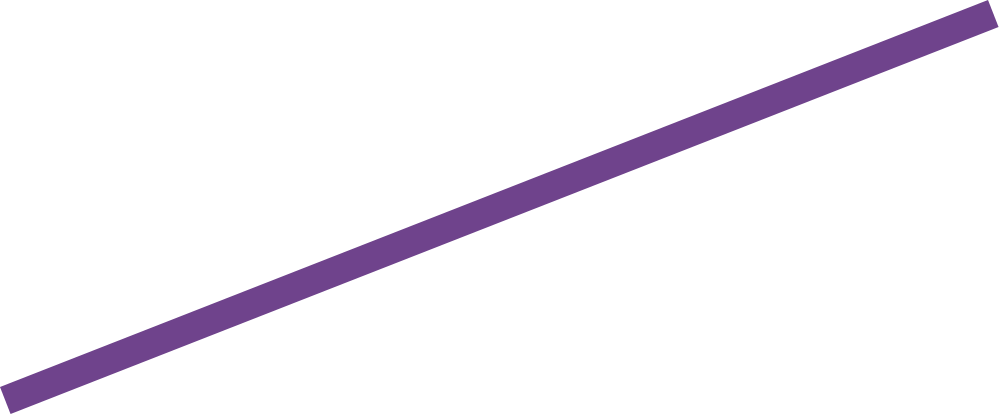
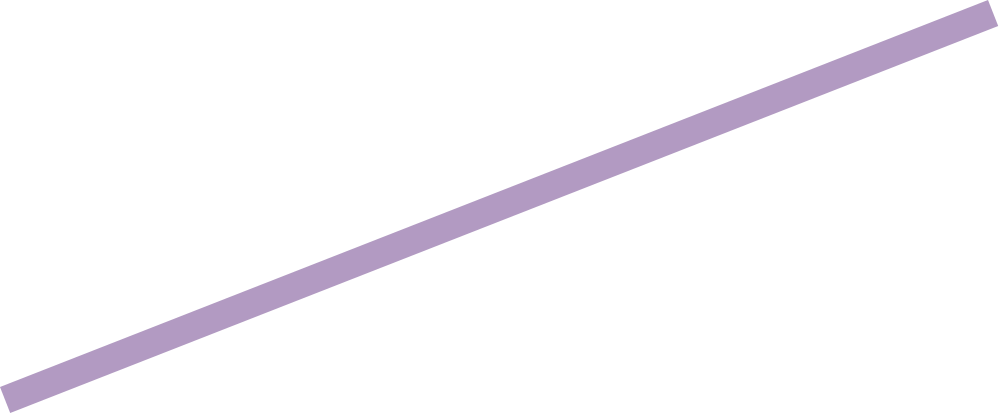


Combining Data Mining and Machine Learning for Effective User Profiling
- Tom Fawcett
- Foster Provost
- Venue: Second International Conference on Knowledge Discovery and Data Mining (KDD96)
- 1996
- Type: Selected Conference Paper
- Acceptance rates: 20%