The goal of causal classification is to identify individuals whose outcome would be positively changed by a treatment. Examples include targeting advertisements and targeting retention incentives to reduce churn. Causal classification is challenging because we observe individuals under only one condition (treated or untreated), so we do not know who was influenced by the treatment, but we may estimate the potential outcomes under each condition to decide who to treat based on treatment effects. Curiously, we often see practitioners using simple outcome prediction instead, eg, will someone purchase if shown the ad? Rather than disregarding this as naive behavior, we undertake a theoretical analysis comparing treatment effect estimation and outcome prediction when addressing causal classification. We find a causal bias-variance tradeoff: because treatment effect estimation depends on two outcome estimates, its larger variance may lead to more errors than the (biased) outcome prediction approach. Large-scale simulations illustrate settings in which outcome prediction should be better, including cases where (1) data to estimate counterfactuals are limited, (2) bias is uniform across individuals, (3) outcomes and treatment effects are positively correlated, and (4) predictions are far from the decision boundary.
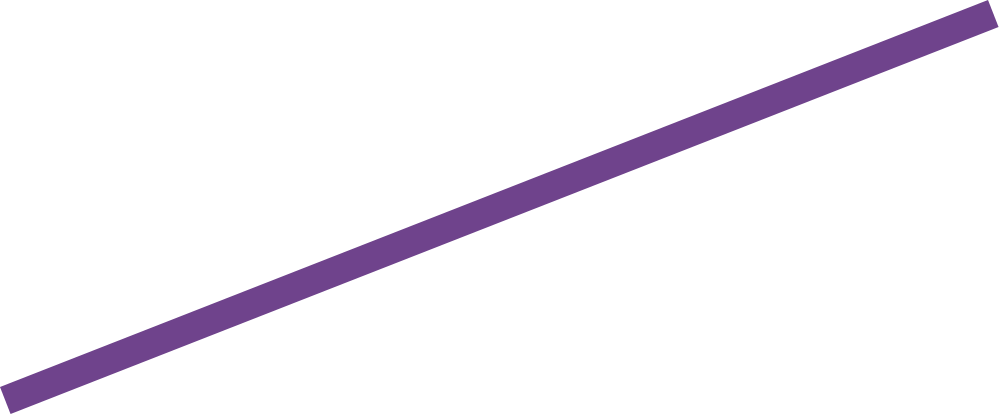
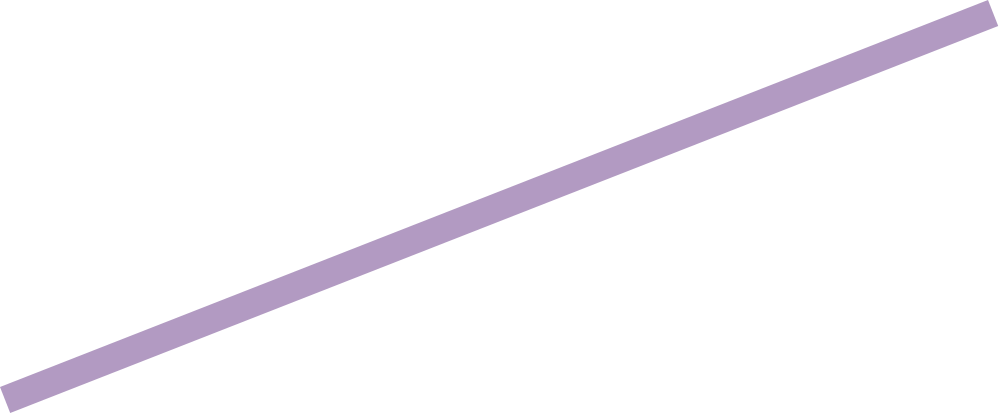


Causal Classification: Treatment Effect Estimation vs. Outcome Estimation
- Carlos Fernández
- Foster Provost
- Venue: Journal of Machine Learning Research
- 2022
- Status: Refereed
- Type: Journal Article