This paper surveys work from the field of machine learning on the problem of within-network learning and inference. To give motivation and context to the rest of the survey, we start by presenting some (published) applications of within-network inference. After a brief formulation of this problem and a discussion of probabilistic inference in arbitrary networks, we survey machine learning work applied to networked data, along with some important predecessors—mostly from the statistics and pattern recognition literature. We then describe an application of within-network inference in the domain of suspicion scoring in social networks. We close the paper with pointers to toolkits and benchmark data sets used in machine learning research on classification in network data. We hope that such a survey will be a useful resource to workshop participants, and perhaps will be complemented by others.
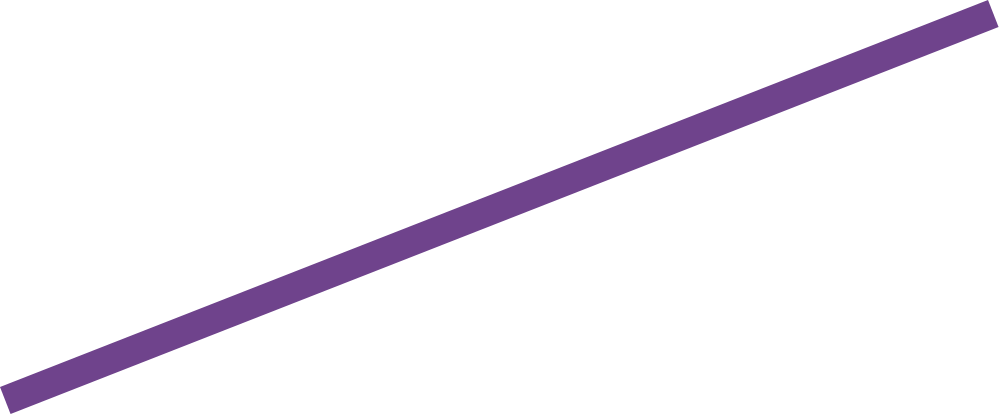
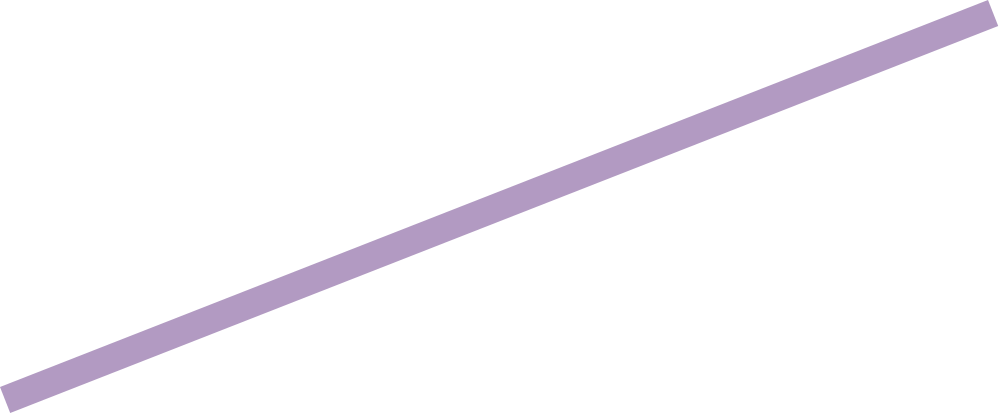


A Brief Survey of Machine Learning Methods for Classification in Networked Data and an Application to Suspicion Scoring
- Sofus Macskassy
- Foster Provost
- Venue: ICML 2006 Workshop
- 2006
- Type: Other Workshop/Symposium Paper