The emergence of online paid micro-crowdsourcing platforms, such as Amazon Mechanical Turk, allows on-demand and at-scale distribution of tasks to human workers around the world. In such settings, online workers come and complete small tasks posted by employers, working for as long or as little as they wish, a process that eliminates the overhead of hiring (and dismissal). This flexibility introduces a different set of inefficiencies: verifying the quality of every submitted piece of work is an expensive operation that often requires the same level of effort as performing the task itself. A number of research challenges arise in such settings. How can we ensure that the submitted work is accurate? What allocation strategies can be employed to make the best use of the available labor force? How can we appropriately assess the performance of individual workers? In this paper, we consider labeling tasks and develop a comprehensive scheme for managing the quality of crowd labeling: First, we present several algorithms for inferring the true classes of objects and the quality of participating workers, assuming the labels are collected all at once before the inference. Next, we allow employers to adaptively decide which object to assign to the next arriving worker and propose several heuristic-based dynamic label allocation strategies to achieve the desired data quality with significantly fewer labels. Experimental results on both simulated and real data confirm the superior performance of the proposed allocation strategies over other existing policies. Finally, we introduce two novel metrics that can be used to objectively rank the performance of crowdsourced workers after fixing correctable worker errors and taking into account the costs of different classification errors. In particular, the worker value metric directly measures the monetary value contributed by each label of a worker toward meeting the quality requirements and provides a basis for the design of fair and efficient compensation schemes.
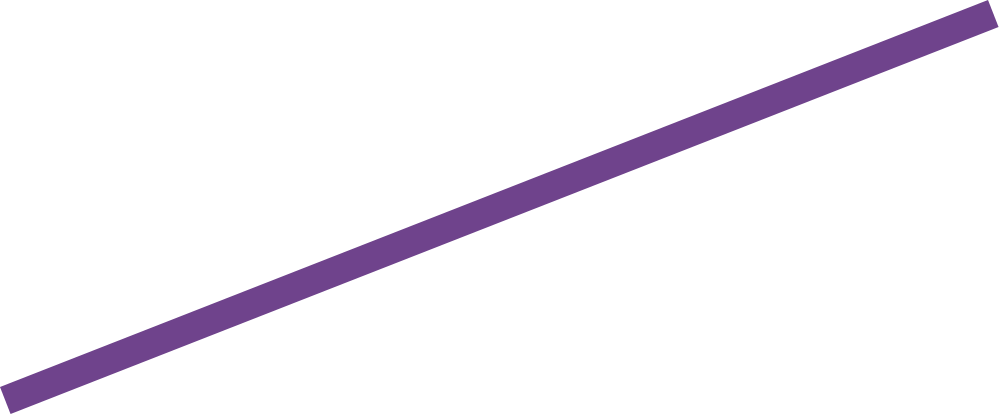
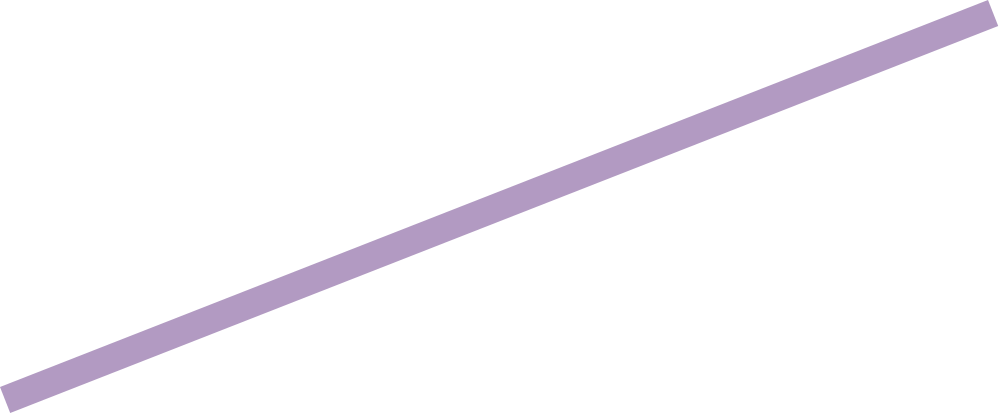


Cost-Effective Quality Assurance in Crowd Labeling
- Panagiotis Ipeirotis
- Foster Provost
- Jing Wang
- Venue: Information Systems Research
- 2017
- Type: Journal Article