The predictive power in ubiquitous big, behavioral data has been emphasized by previous academic research. The ultra-high dimensional and sparse characteristics, however, pose significant challenges on state-of-the-art classification techniques. Moreover, no consensus exists regarding a feasible trade-off between classification performance and computational complexity. This work provides a contribution in this direction through a systematic benchmarking study. Forty-three fine-grained behavioral data sets are analyzed with 11 classification techniques. Statistical performance comparisons enriched with learning curve analyses demonstrate two important findings. Firstly, an inherent AUC-time trade-off becomes clear, making the choice for an appropriate classifier dependent on time restrictions and data set characteristics. Logistic regression achieves the best AUC, however in the worst amount of time. Also, L2 regularization proves better than sparse L1-regularization. An attractive trade-off is found in a similarity-based technique called PSN. Secondly, the results illustrate that significant value lies in collecting and analyzing even more data, both in the instance and in the feature dimension, contrasting findings on traditional data. The results of this study provide guidance for researchers and practitioners for the selection of appropriate classification techniques, sample sizes and data features, while also providing focus in scalable algorithm design in the face of large, behavioral data.
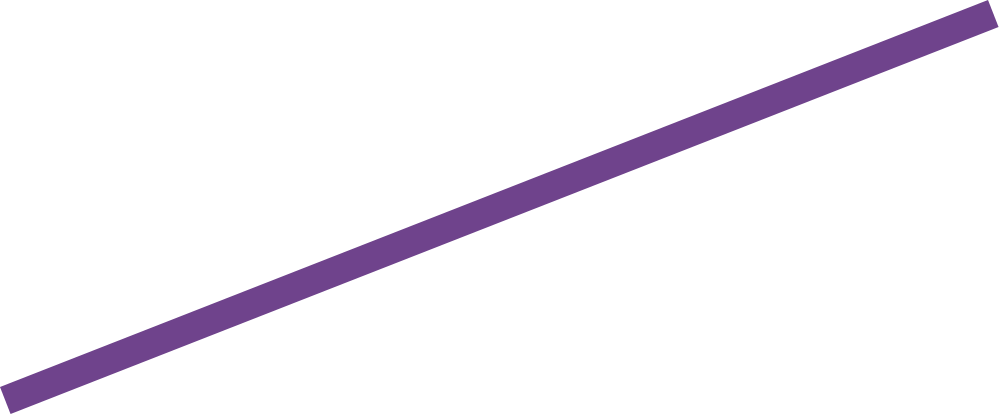
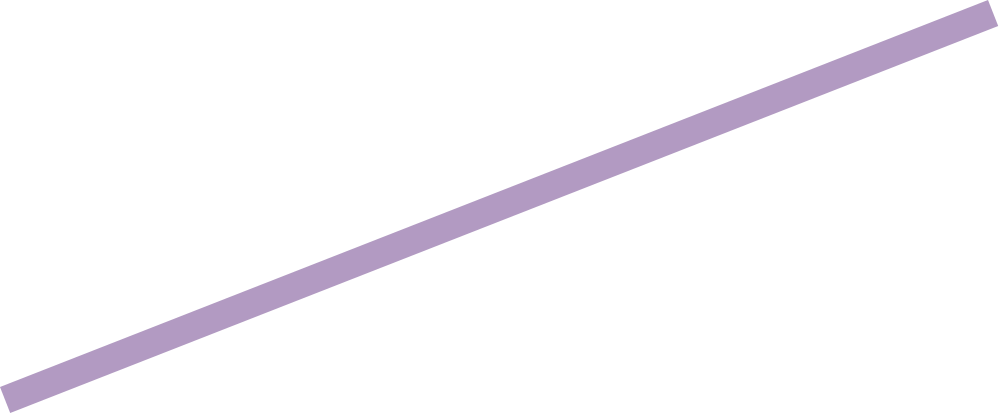


A Benchmarking Study of Classification Techniques for Behavioral Data
- Sofie De Cnudde
- David Martens
- Theodoros Evgeniou
- Foster Provost
- Venue: Int J Data Sci Analytics
- 2020
- Status: Refereed
- Type: Journal Article